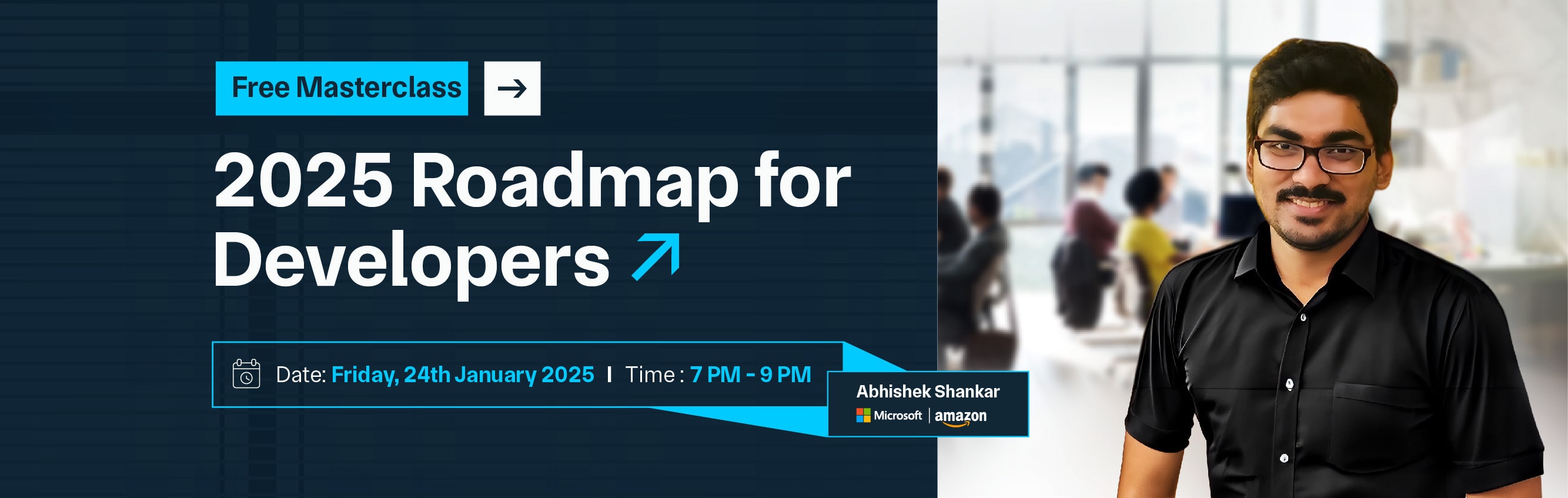

Pooling in Convolutional Neural Networks (CNNs) is a crucial operation used to reduce the spatial dimensions of feature maps, thereby decreasing computational load and the number of parameters. This process simplifies the network, helps prevent overfitting, and retains essential features for further processing. The most common pooling techniques are max pooling and average pooling.
Max pooling selects the maximum value from a set of values within a specific window, while average pooling computes the average value. Typically, pooling is applied using a window or kernel of size 2x2 or 3x3, which strides over the feature map, reducing its dimensions by aggregating information. By performing this down-sampling, pooling layers help maintain the most critical features while discarding less important information, leading to more robust and generalized models.
Pooling also aids in making the network more invariant to small translations and distortions, enhancing its ability to recognize patterns regardless of their location within the image. Overall, pooling is a fundamental technique in CNNs that contributes to efficient and effective deep learning architectures.
Pooling in Convolutional Neural Networks (CNNs) is a technique used to downsample feature maps, thereby reducing their spatial dimensions while preserving the essential information.
This operation simplifies the representation of data, reduces the computational burden, and helps the network generalize better to unseen data. Here's a detailed breakdown:
Pooling is a process applied to the output of convolutional layers to decrease the feature map's spatial size. It involves sliding a pooling window (e.g., 2x2) over the feature map and applying a pooling function to the values within this window. The pooling function aggregates information from each window to produce a smaller, summarized output.
Pooling layers are used in Convolutional Neural Networks (CNNs) for several important reasons:
Overall, pooling layers play a key role in enhancing the efficiency, robustness, and effectiveness of CNNs.
Pooling layers in Convolutional Neural Networks (CNNs) come in several types, each with its specific method of aggregating features. Here are the most commonly used types:
This method selects the maximum value from a set of values within a specified window or kernel. For instance, in a 2x2 max pooling operation, the window scans through the feature map, and only the maximum value within each window is retained. Max pooling helps retain the most prominent features and is effective in capturing the most critical aspects of the data.
Code:
import numpy as np
import tensorflow as tf
from tensorflow.keras.layers import MaxPooling2D
# Define a 4x4 input matrix
input_matrix = np.array([[1, 3, 2, 4],
[5, 6, 7, 8],
[9, 10, 11, 12],
[13, 14, 15, 16]], dtype=float)
# Reshape for a single channel and batch dimension
input_matrix = input_matrix.reshape((1, 4, 4, 1))
# Apply MaxPooling2D
max_pooling = MaxPooling2D(pool_size=(2, 2))
output = max_pooling(tf.convert_to_tensor(input_matrix))
print("Max Pooling Output:")
print(output.numpy().reshape(2, 2))
Output:
Max Pooling Output:
[[ 6. 8.]
[14. 16.]]
Average pooling computes the average value within the pooling window. Unlike max pooling, which emphasizes the most significant feature, average pooling provides a smoother, more generalized representation of the features by averaging all values in the window. This can be useful in scenarios where a less aggressive approach to feature extraction is desired.
Code:
from tensorflow.keras.layers import AveragePooling2D
# Apply AveragePooling2D
average_pooling = AveragePooling2D(pool_size=(2, 2))
output = average_pooling(tf.convert_to_tensor(input_matrix))
print("Average Pooling Output:")
print(output.numpy().reshape(2, 2))
Output:
Average Pooling Output:
[[ 3.5 5.5]
[11.5 13.5]]
Instead of applying pooling to local regions, global average pooling computes the average value of the entire feature map. This reduces the feature map to a single value per channel, effectively summarizing the spatial information into a compact representation. It’s often used before the final classification layer in CNN architectures.
Code:
from tensorflow.keras.layers import GlobalAveragePooling2D
# Define the layer
global_avg_pooling = GlobalAveragePooling2D()
output = global_avg_pooling(tf.convert_to_tensor(input_matrix))
print("Global Average Pooling Output:")
print(output.numpy())
Output:
Global Average Pooling Output:
[ 7.5]
Similar to global average pooling, global max pooling takes the maximum value from the entire feature map, compressing it into a single value per channel. This approach captures the most significant feature across the entire spatial domain of the feature map.
Code:
from tensorflow.keras.layers import GlobalMaxPooling2D
# Define the layer
global_max_pooling = GlobalMaxPooling2D()
output = global_max_pooling(tf.convert_to_tensor(input_matrix))
print("Global Max Pooling Output:")
print(output.numpy())
Output:
Global Max Pooling Output:
[16.]
Although less common, min pooling selects the minimum value within a pooling window. This technique is used less frequently but can be useful in specific applications where capturing the least prominent features is beneficial.
Code:
import tensorflow as tf
from tensorflow.keras.layers import Layer
class MinPooling2D(Layer):
def __init__(self, pool_size=(2, 2), **kwargs):
super(MinPooling2D, self).__init__(**kwargs)
self.pool_size = pool_size
def call(self, inputs):
return tf.nn.pool(inputs, window_shape=self.pool_size, pooling_type='MIN', padding='VALID')
# Apply MinPooling2D
min_pooling = MinPooling2D(pool_size=(2, 2))
output = min_pooling(tf.convert_to_tensor(input_matrix))
print("Min Pooling Output:")
print(output.numpy().reshape(2, 2))
Output:
Min Pooling Output:
[[1. 2.]
[9. 10.]]
Fractional pooling allows for pooling with non-integer window sizes and strides, providing more flexibility in down-sampling operations. This can help in scenarios where standard pooling methods may be challenging.
Fractional pooling typically requires a specialized library or custom implementation beyond basic TensorFlow/Keras functionality. Each type of pooling layer has its advantages and is chosen based on the specific requirements of the CNN architecture and the problem being addressed.
Pooling is a technique used in Convolutional Neural Networks (CNNs) to down-sample feature maps, which helps to reduce the dimensionality of the data, making the network more efficient and robust. Here’s a detailed explanation of how pooling works:
Pooling operations work by sliding a window or kernel over the input feature map and applying a specific aggregation function to the values within that window. The most common pooling operations are max pooling and average pooling, but there are other variations as well.
Here's a step-by-step breakdown of the pooling process:
Pooling layers are components of Convolutional Neural Networks (CNNs) designed to reduce the spatial dimensions of feature maps, thereby simplifying the network and improving computational efficiency. They are essential in deep learning architectures for image processing and other tasks involving spatial data. Here’s a detailed overview:
Pooling layers play a vital role in Convolutional Neural Networks (CNNs) and are used to address several key challenges in the training and functioning of these networks. Here’s a detailed look at their use and benefits:
Purpose: Pooling layers significantly reduce the spatial dimensions of feature maps. This reduction is crucial for several reasons:
Example: A 4x4 feature map with a 2x2 max pooling layer will be reduced to a 2x2 feature map. This reduction simplifies the data and makes it more manageable for the network.
Purpose: Pooling layers help in extracting and retaining important features from the input data. They provide a way to:
Example: Max pooling captures the most prominent features by selecting the maximum value in each pooling window, which can be critical for identifying strong features in an image.
Purpose: Pooling layers contribute to making CNNs invariant to small translations and distortions in the input data:
Example: An object detected in one part of an image will be recognized in another part due to the pooling layer’s ability to handle slight variations in position.
Purpose: Pooling layers help in reducing noise and variations in the input data:
Example: Average pooling smooths out variations in the feature map by averaging values, which can help in reducing the impact of noise or small perturbations in the input.
Purpose: Pooling layers facilitate hierarchical feature learning by progressively reducing the spatial dimensions of feature maps:
Example: In deep CNN architectures, pooling layers at various stages helps in progressively capturing higher-level features from the original input image, such as shapes, textures, and objects.
Pooling layers offer several advantages in Convolutional Neural Networks (CNNs) that significantly enhance their performance and efficiency. Here’s a detailed look at the key benefits:
Advantage: Pooling layers reduce the spatial dimensions of feature maps, which has several benefits:
Example: Reducing a 64x64 feature map to 32x32 through max pooling can drastically cut down on the number of parameters and operations in later layers.
Advantage: Pooling helps in summarizing and retaining the most significant features from the input data:
Example: Max pooling retains the most prominent feature in a region, such as the strongest edge in an image, which is important for object recognition.
Advantage: Pooling layers make CNNs more robust to small translations and distortions:
Example: An object detected in one part of an image will still be recognized even if it moves slightly to another part of the image due to pooling’s ability to handle such shifts.
Advantage: Pooling layers help in smoothing out noise and variations in the input data:
Example: Average pooling smooths out variations by averaging values, which helps in reducing the impact of noisy or inconsistent pixels.
Advantage: Pooling facilitates hierarchical feature learning by progressively abstracting the feature maps:
Example: In a deep CNN, pooling layers at different levels help in capturing low-level features like edges and high-level features like shapes and objects.
Advantage: Pooling layers contribute to simpler network designs by reducing the number of parameters and layers needed:
Example: Using pooling layers allows for building deeper networks that can learn more complex patterns without a proportional increase in the number of parameters.
Advantage: Pooling layers can contribute to faster convergence during training:
Example: Pooling reduces the number of calculations and parameters, which can lead to faster convergence of the network during the training phase.
While pooling layers offer several advantages in Convolutional Neural Networks (CNNs), they also come with some disadvantages. Here’s a detailed look at the potential drawbacks of using pooling layers:
Disadvantage: Pooling layers can lead to a loss of spatial information:
Example: In an image classification task, max pooling might discard subtle but important features that differentiate between similar classes.
Disadvantage: Pooling reduces the spatial resolution of feature maps:
Example: In object detection, losing spatial resolution might make it harder for the network to pinpoint the exact location of objects in an image.
Disadvantage: Pooling provides translation invariance but can sometimes reduce the network’s ability to discriminate between similar features:
Example: In a facial recognition system, pooling might blur distinctions between facial features, making it harder to differentiate between similar faces.
Disadvantage: Pooling layers use fixed, non-learnable operations:
Example: Fixed pooling might not capture complex patterns as effectively as learned pooling strategies or alternative methods.
Disadvantage: Pooling layers can cause issues during backpropagation:
Example: In a deep network, the pooling layers might cause gradient bottlenecks, impacting the overall learning process.
Disadvantage: Pooling layers provide limited flexibility in feature extraction:
Example: For images with varying resolutions or scales, fixed pooling parameters might not be optimal for all regions of the image.
Disadvantage: Other techniques might offer benefits over traditional pooling:
Example: In modern architectures like ResNet or EfficientNet, strided convolutions or adaptive pooling might be used instead of traditional pooling layers.
Pooling layers are essential in Convolutional Neural Networks (CNNs), offering significant advantages such as dimensionality reduction, feature extraction, translation invariance, and noise reduction. By reducing the spatial dimensions of feature maps, pooling layers decrease computational costs and memory usage while retaining key features and providing robustness to small translations and distortions. They also help smooth out noise and support hierarchical feature learning by summarizing local regions of the input data.
However, pooling layers come with drawbacks, including potential loss of detailed spatial information, reduced resolution that can affect precise localization tasks, and decreased discriminative ability due to the fixed nature of their operations. Additionally, the non-differentiable nature of pooling can complicate gradient flow during backpropagation. Despite these limitations, pooling remains a valuable tool in CNN architectures. Advances such as strided convolutions and adaptive pooling offer alternatives that can address some of these challenges, leading to more effective and efficient deep learning models. Balancing these benefits and drawbacks is key to designing CNNs that perform well across various tasks.
Copy and paste below code to page Head section
A pooling layer in a CNN is a layer that reduces the spatial dimensions of the feature maps while retaining the most important information. It aggregates values from local regions of the feature map using operations like max pooling or average pooling, which helps in simplifying the data and making the network more computationally efficient.
Pooling is important because it helps in reducing the dimensionality of feature maps, which decreases computational requirements and memory usage. It also makes the network more robust to small translations and distortions in the input data and helps in extracting key features while smoothing out noise.
Max pooling selects the highest value from each region of the feature map, highlighting the most prominent features. Average pooling, on the other hand, computes the average of the values in each region, which can smooth out features and reduce sensitivity to noise. Max pooling generally retains stronger features, while average pooling can provide a more generalized representation.
Pooling affects training by reducing the size of feature maps, which speeds up computation and reduces memory usage. This allows for deeper networks and faster convergence. However, it can also impact performance by losing spatial details and potentially reducing the network's ability to discriminate between fine details.
Yes, alternatives to traditional pooling layers include: Strided Convolutions: Use convolutional layers with a stride greater than 1 to down-sample the feature maps while learning features. Adaptive Pooling: Allows pooling sizes to be adjusted dynamically based on the input dimensions or requirements. Global Average Pooling: Reduces the entire feature map to a single value per channel, which can be useful for classification tasks.
Pooling layers are beneficial in CNNs when you want to reduce the dimensionality of feature maps, decrease computational requirements, and make the network more robust to small translations and distortions. They are particularly useful in early layers of the network to manage computational complexity and emphasize important features.