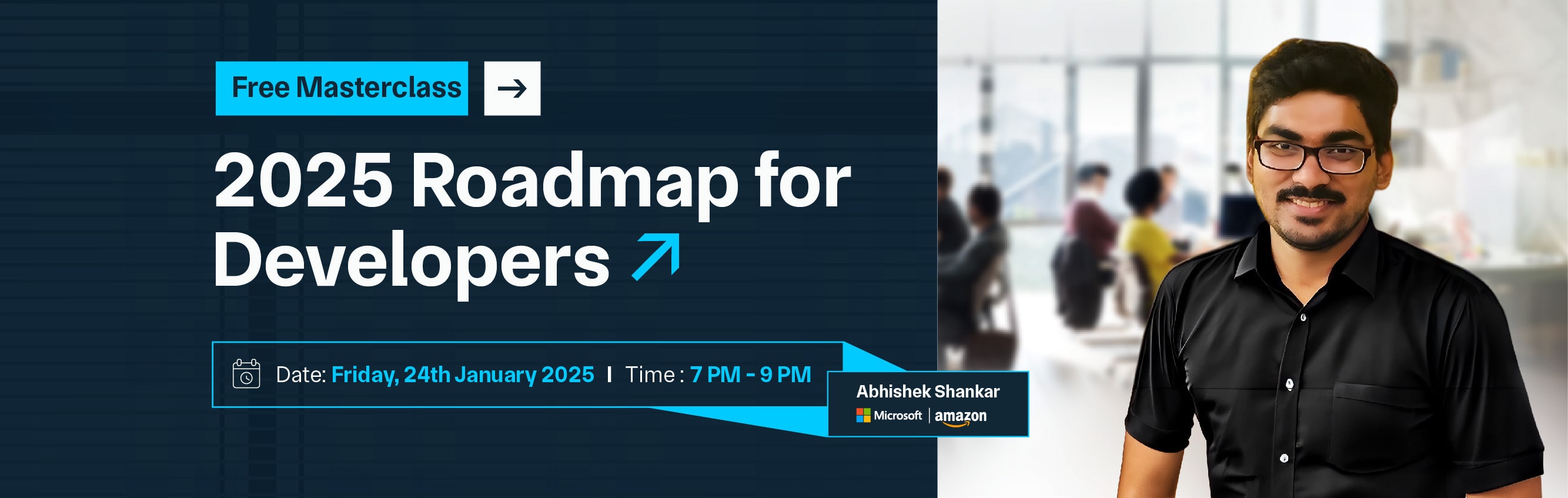

Big Data, while offering substantial benefits, also comes with its own set of challenges and disadvantages. One primary concern is the immense volume of data that organizations must handle. Managing and processing such large datasets often requires advanced infrastructure, including high-performance hardware and complex software solutions. This necessity can lead to significant costs and complexity, particularly for smaller organizations that may need help with the financial and technical demands of Big Data systems. Another critical issue is data privacy and security.
As organizations gather vast amounts of sensitive information, they become prime targets for cyberattacks. Ensuring robust security measures and compliance with data protection regulations is essential, but it can also be incredibly challenging. Data breaches can result in severe legal consequences, financial losses, and reputational damage, making data security a top priority for businesses dealing with Big Data.
Furthermore, the analysis of Big Data can sometimes yield misleading or incorrect insights if not executed properly. Issues such as data quality, including inaccuracies or incompleteness, can lead to flawed conclusions and poor decision-making. To mitigate these risks, organizations must invest in stringent data governance practices to ensure data accuracy and reliability, adding to the complexity and resource requirements of managing Big Data effectively.
Big Data refers to the vast and complex sets of data that organizations generate and collect from various sources. Unlike traditional data, Big Data encompasses enormous volumes of information that are too large or complex for conventional data-processing tools to handle efficiently. This data comes from diverse sources such as social media, sensors, transaction records, and more.
The three key characteristics of Big Data are its volume (the sheer amount of data), velocity (the speed at which data is generated and processed), and variety (the different types and formats of data). The primary goal of Big Data is to extract valuable insights and patterns that can inform business decisions and drive strategic actions.
By leveraging advanced analytics and machine learning techniques, organizations can analyze these massive datasets to uncover trends, predict future outcomes, and enhance decision-making. Despite its potential, managing Big Data presents challenges, including the need for substantial storage and processing resources, as well as ensuring data quality and security. Properly harnessed, Big Data can provide a competitive edge and fuel innovation across various industries.
Organizations can leverage Big Data to gain a competitive edge and drive business success in numerous ways. By analyzing large datasets, they can uncover valuable insights into customer behavior, market trends, and operational efficiencies. This information allows for more informed decision-making, targeted marketing strategies, and personalized customer experiences.
For instance, businesses can use Big Data to optimize their supply chain by predicting demand patterns and managing inventory more effectively. In marketing, Big Data helps in understanding customer preferences and creating targeted campaigns that improve engagement and conversion rates. Additionally, organizations can employ Big Data analytics to enhance product development, improve service delivery, and streamline operations.
Moreover, Big Data can be used for advanced predictive analytics, identifying potential risks and opportunities before they arise. It also enables organizations to track performance metrics in real-time, allowing for quicker adjustments and more agile responses to changing conditions. By integrating Big Data into their strategic processes, organizations can drive innovation, increase efficiency, and maintain a competitive advantage in their respective markets.
Big Data offers a multitude of advantages that can transform how organizations operate and compete. By analyzing extensive datasets, businesses can uncover patterns, gain actionable insights, and drive strategic decisions. The ability to process and interpret vast amounts of data enables companies to improve efficiency, enhance customer experiences, and foster innovation.
This powerful tool not only optimizes internal processes but also provides a competitive edge in the marketplace. Leveraging Big Data can lead to significant advancements in various domains, from marketing and operations to finance and product development.
Big Data enhances decision-making by offering a comprehensive view of information that goes beyond intuition. Access to large datasets allows organizations to make informed choices based on concrete evidence rather than guesswork.
This data-driven approach improves forecasting accuracy, risk assessment, and strategic planning. By analyzing trends and patterns, businesses can align decisions with their goals and market demands, resulting in more effective strategies and better outcomes.
With Big Data, organizations can delve deeply into customer behavior, preferences, and purchasing patterns. By analyzing diverse data sources, such as social media, transaction records, and feedback, businesses can gain a nuanced understanding of their customers.
This insight enables companies to tailor their offerings more precisely, enhancing customer satisfaction and loyalty. Personalized products, services, and experiences that meet individual needs become achievable, leading to a stronger customer relationship.
Big Data provides organizations with a significant competitive edge by offering insights that may not be available to competitors. By analyzing market trends, consumer behavior, and competitive dynamics, businesses can identify opportunities and make proactive decisions.
This strategic advantage helps companies stay ahead of market changes, foster innovation, and develop unique strategies. Leveraging data-driven insights allows businesses to maintain a strong position in their industry and respond swiftly to emerging trends.
Big Data drives innovation by revealing trends and patterns that can inspire new products and services. Analyzing customer feedback, market trends, and emerging technologies helps organizations identify gaps in the market and develop solutions to address unmet needs.
This approach fosters a culture of innovation, enabling businesses to create offerings that align with evolving consumer demands. Staying ahead of competitors becomes possible through continuous product development and market responsiveness.
Big Data enhances effective risk management through the use of predictive analytics and trend analysis. By examining historical data and identifying potential risk factors, organizations can anticipate and mitigate risks before they become critical issues.
This proactive approach helps manage uncertainties, protect against potential threats, and make informed decisions. Safeguarding assets and reputation is achieved by addressing risks in advance, ensuring business continuity.
Big Data facilitates real-time analytics, enabling organizations to make immediate decisions based on up-to-date information. This capability is crucial for responding to dynamic market conditions and monitoring performance metrics.
Real-time analytics enhances agility and responsiveness, allowing businesses to capitalize on emerging opportunities and address challenges as they arise. Swift adaptation and decision-making are made possible, improving overall operational efficiency.
Big Data improves supply chain management by offering insights into inventory levels, demand forecasts, and supplier performance. Analyzing this data allows organizations to optimize inventory management, reduce lead times, and enhance logistics.
Improved supply chain management leads to cost savings, increased efficiency, and better alignment with customer demands. Effective resource allocation and streamlined processes result in a more responsive and efficient supply chain.
Big Data enhances fraud detection through the analysis of patterns and anomalies within large datasets. Advanced analytics can identify suspicious activities and potential fraud indicators, enabling organizations to implement preventive measures.
This capability helps protect against financial losses and maintains the integrity of business operations. Effective fraud detection systems reduce the risk of fraudulent activities and ensure a secure operational environment.
Personalization is greatly enhanced by Big Data, allowing businesses to tailor interactions with customers based on detailed insights. Understanding individual preferences and behaviors enables organizations to deliver personalized experiences, targeted promotions, and relevant content.
This level of personalization strengthens customer engagement, drives loyalty, and improves overall satisfaction. Tailoring services and communication to individual needs leads to a more meaningful customer relationship.
Big Data supports strategic planning by providing valuable insights into market trends, customer needs, and competitive dynamics. Analyzing this data helps organizations develop effective strategies, set realistic goals, and make informed decisions.
Data-driven strategic planning ensures alignment with market demands and long-term success. Businesses can navigate complex environments and achieve their objectives with greater confidence and precision.
In sectors such as healthcare and manufacturing, Big Data contributes to health and safety improvements by analyzing data related to workplace conditions, equipment performance, and patient outcomes.
This analysis helps identify potential hazards, implement safety measures, and enhance protocols. Improved health and safety protocols lead to a safer work environment and better health outcomes, reducing risks and ensuring compliance with safety regulations.
Big Data enhances marketing effectiveness by providing insights into customer behavior, campaign performance, and market trends. This data-driven approach enables organizations to optimize marketing strategies, improve targeting, and achieve better results.
Enhanced marketing effectiveness translates to increased brand visibility, higher conversion rates, and better ROI. Data-driven marketing decisions lead to more successful campaigns and a stronger market presence.
While big data offers numerous benefits, it also presents several challenges and disadvantages that organizations must navigate. The sheer volume and complexity of data can lead to issues such as data quality and integration difficulties.
Additionally, the costs associated with storing and processing large datasets can be substantial, especially for smaller businesses. Privacy and security concerns also arise as organizations handle sensitive customer information.
Moreover, there is a risk of information overload, where decision-makers need help to extract meaningful insights from vast data pools. These disadvantages highlight the need for careful planning and implementation when leveraging big data in business operations.
One significant disadvantage of big data is the issue of data quality and accuracy. With the vast amount of data collected from various sources, ensuring that the data is clean, consistent, and reliable can be challenging. Only accurate or complete data can lead to accurate conclusions and good decision-making.
For instance, in retail, if customer purchase data needs to be properly validated, it can result in misguided marketing strategies or inventory management decisions. Therefore, organizations need to implement robust data validation and cleansing processes to maintain data integrity and avoid costly mistakes.
Integrating big data from different sources can be a complex task, especially when dealing with structured and unstructured data types. Organizations often collect data from multiple channels, such as social media, online transactions, and customer interactions, which may use different formats and standards.
The lack of standardization makes it difficult to integrate and analyze data seamlessly, potentially leading to fragmented insights and inefficiencies. In the retail industry, for example, integrating data from physical stores and online platforms requires sophisticated systems and expertise to ensure a holistic view of customer behavior.
The costs associated with big data infrastructure and analytics tools can be a significant barrier, especially for smaller businesses. Storing, processing, and analyzing large datasets require substantial investments in technology, hardware, and skilled personnel. In retail, companies may need to invest in advanced analytics platforms and hire data scientists to derive meaningful insights from customer data.
Additionally, the ongoing maintenance and upgrading of these systems can add to the expenses. Therefore, organizations must carefully weigh the potential benefits against the costs and ensure that their big data initiatives align with their budget and strategic goals.
Handling large volumes of sensitive customer information raises significant privacy and security concerns. Big data often involves collecting and storing personal data, such as names, addresses, and purchasing habits, which cybercriminals can target. Retail businesses, in particular, must safeguard customer data to prevent data breaches and maintain trust.
Compliance with data protection regulations, such as GDPR, is crucial to avoid legal repercussions and financial penalties. Implementing robust security measures, including encryption and access controls, is essential to protect data privacy and ensure that customer information remains secure.
Another challenge of big data is the risk of information overload, where decision-makers are overwhelmed by the sheer volume of data available. With so much information to process, it can take time to identify the most relevant and actionable insights. In retail, for instance, managers may need help to discern meaningful trends in customer behavior amidst the noise of vast data points.
This can lead to analysis paralysis, where decision-making is delayed or hindered by the overwhelming amount of information. To mitigate this risk, organizations need to develop effective data management and analytics strategies that focus on extracting value from data rather than quantity.
Data silos occur when data is isolated within departments or systems, making it difficult to share and collaborate across an organization. In large companies, different departments use separate databases or platforms that need to be more easily integrated, leading to inefficiencies and miscommunication. In retail, for example, marketing, sales, and supply chain departments may have disparate data systems, hindering a unified customer experience.
Breaking down these silos requires a comprehensive data strategy that encourages collaboration and data sharing across the organization to ensure that all departments have access to relevant and consistent data.
Managing big data involves dealing with complex datasets that require advanced tools and expertise to process and analyze. This complexity can pose a significant challenge for organizations, particularly those needing more technical skills or resources. In retail, companies may need help managing large volumes of customer data, requiring sophisticated data management systems and personnel with expertise in data analytics.
This complexity can also slow down data processing times and hinder the ability to gain timely insights, making it essential for organizations to invest in the right tools and training for their teams.
As data volumes grow, organizations may face scalability challenges with their existing infrastructure. Traditional data processing systems may need help to handle the increasing data loads efficiently, leading to performance bottlenecks and delays. In retail, the growing volume of transaction data from online and offline channels can strain existing systems, impacting the ability to provide timely insights and customer service.
To address scalability issues, organizations need to invest in scalable big data solutions that can handle the demands of growing data sets, ensuring smooth operations and continued data-driven decision-making.
Maintaining data consistency is crucial for accurate analysis and decision-making. However, with big data, ensuring consistency across different data sources and systems can be challenging. Inconsistent data can lead to conflicting insights and hinder the ability to make informed decisions.
For retail companies, consistent pricing, product, or inventory data across different platforms can positively impact customer experience and operational efficiency. Implementing data governance policies and procedures is essential to ensure that data remains consistent and reliable across the organization, enabling accurate analysis and insights.
The effective use of big data requires a significant reliance on technology and skilled personnel. Organizations must invest in advanced analytics tools, data processing platforms, and skilled data scientists to extract meaningful insights from large datasets. This dependency on technology and expertise can be a disadvantage, especially for smaller businesses with limited resources.
In retail, companies need to rely on sophisticated systems to analyze customer data and optimize operations, making it crucial to have access to the right tools and personnel. This reliance on technology can also make organizations vulnerable to disruptions in case of technical failures or loss of skilled staff.
Big data has the potential to revolutionize both organizations and society by providing insights that drive innovation, efficiency, and improvement in various fields. By leveraging big data, businesses can enhance decision-making, optimize operations, and create personalized customer experiences.
In society, big data can address critical issues like healthcare, environmental sustainability, and urban planning. By analyzing large datasets, organizations can uncover patterns and trends that inform strategic initiatives and public policies.
The effective use of big data can lead to smarter solutions, improved quality of life, and a more sustainable future. Here are some key ways big data can be used to benefit organizations and society:
By effectively harnessing the power of big data, organizations can drive positive change, improve efficiency, and create value for both themselves and society at large.
Big data encompasses a wide variety of data types generated from diverse sources. Understanding these different types is crucial for organizations looking to harness the power of big data effectively.
Generally, big data can be categorized into three main types: structured, unstructured, and semi-structured data. Each type has its characteristics, storage requirements, and methods of analysis.
Structured data is highly organized and easily searchable, while unstructured data is more complex and less organized. Semi-structured data falls between the two, with some organizational properties. By understanding these types of data, organizations can better utilize big data to drive insights and innovation.
Structured data refers to data that is highly organized and easily searchable in a fixed format, such as tables or spreadsheets. It is stored in relational databases and is characterized by its well-defined schema, which makes it straightforward to query and analyze. Common examples of structured data include customer information, transaction records, and inventory data.
Businesses often use SQL (Structured Query Language) to manage and analyze structured data. This type of data is essential for operations that require precise and consistent information, such as financial transactions and inventory management.
Unstructured data is data that needs a predefined structure or schema, making it more complex to process and analyze. It includes a wide variety of data formats, such as text, images, videos, social media posts, emails, and audio files. Unstructured data is generated from various sources, including social media platforms, customer reviews, and multimedia content.
Due to its complexity and lack of uniformity, analyzing unstructured data requires advanced analytics tools and techniques, such as natural language processing (NLP) and machine learning algorithms, to extract meaningful insights.
Semi-structured data is a type of data that contains elements of both structured and unstructured data. It needs to fit neatly into a relational database. Still, it contains some organizational properties, such as tags or markers, that make it easier to parse and analyze than fully unstructured data.
Examples of semi-structured data include XML files, JSON documents, and log files. This data type is commonly used in web applications and data interchange formats. Analyzing semi-structured data often involves tools that can recognize and interpret the embedded tags and markers to extract useful information.
Machine-generated data refers to data created automatically by machines without human intervention. This includes data from sensors, IoT devices, server logs, industrial equipment, and scientific instruments. Machine-generated data is typically produced at high velocity and in large volumes, making it a significant component of big data.
It is often used in industries such as manufacturing, logistics, and healthcare for monitoring and predictive maintenance. Organizations use big data analytics tools to process and analyze machine-generated data, gaining insights into operational efficiencies and potential issues.
Individuals create human-generated data through their interactions with digital platforms and services. This includes data from social media posts, emails, website interactions, and customer feedback. Human-generated data is rich in context and can provide insights into consumer behavior, preferences, and sentiment.
Businesses leverage this data for marketing strategies, customer experience enhancements, and brand reputation management. However, analyzing human-generated data can be challenging due to its variability and the need for sentiment analysis and text-mining techniques.
Spatial data, also known as geospatial data, refers to data that is associated with a specific location on the earth's surface. This data type includes coordinates, addresses, maps, and any information that has a geographical aspect.
Spatial data is commonly used in applications such as geographic information systems (GIS), navigation systems, and urban planning. It enables organizations to analyze location-based patterns and trends, aiding in decision-making processes related to site selection, logistics optimization, and environmental conservation.
Temporal data is data that is time-dependent and involves timestamps to indicate when an event occurred, or a data point was recorded. Examples include stock prices, weather data, website traffic, and transactional data.
Temporal data analysis is crucial for understanding trends, patterns, and seasonality. Businesses use temporal data to forecast demand, analyze market trends, and optimize supply chains. Time series analysis, which examines data points ordered by time, is a common method for analyzing temporal data.
Big data works by capturing, storing, processing, and analyzing vast volumes of data from various sources to extract valuable insights. The process begins with data collection from diverse inputs such as social media, sensors, customer transactions, and online activities. This data is then stored in scalable storage systems like Hadoop or cloud-based solutions, which can handle large datasets efficiently.
Once stored, the data undergoes processing using powerful tools and frameworks, such as Apache Spark or Hadoop MapReduce, to clean, transform, and organize it for analysis. The final step involves analyzing the processed data using advanced analytics techniques, including machine learning algorithms, data mining, and statistical analysis.
This analysis helps organizations uncover patterns, trends, and correlations that inform decision-making and drive innovation. By leveraging big data analytics, businesses can optimize operations, improve customer experiences, and gain a competitive edge in their respective industries.
Working with big data presents numerous challenges that organizations must overcome to harness its potential successfully. As data volumes grow exponentially, managing and processing these massive datasets becomes increasingly complex. Big data often comes from diverse sources and in different formats, requiring sophisticated tools and techniques for integration and analysis.
Ensuring data quality and accuracy is another significant challenge, as poor data quality can lead to incorrect insights and decisions. Additionally, organizations must address concerns related to data privacy and security, as handling sensitive information increases the risk of data breaches.
Big data strategies focus on effectively managing and leveraging large volumes of data to gain valuable insights and drive business outcomes. An effective strategy begins with defining clear objectives and identifying the specific data needs aligned with business goals. This involves selecting appropriate technologies and tools for data storage, processing, and analysis, such as cloud-based platforms, Hadoop, or Apache Spark.
Additionally, organizations should implement robust data governance practices to ensure data quality, security, and compliance with regulations. Developing a scalable architecture that can handle growing data volumes and integrating data from various sources are also key components of a successful strategy. Solutions for big data often involve using advanced analytics techniques and technologies to extract actionable insights from complex datasets. This includes employing machine learning algorithms, data mining, and predictive analytics to uncover patterns and trends.
Data visualization tools play a crucial role in presenting these insights in an understandable format, helping stakeholders make informed decisions. Leveraging real-time analytics can provide immediate insights and enable quick responses to changing conditions. By implementing these solutions, organizations can optimize operations, enhance customer experiences, and drive innovation across their business processes.
Big data is prevalent across various industries, showcasing its versatility and impact. Examples illustrate how organizations harness large and complex datasets to drive decisions, improve efficiency, and enhance customer experiences.
From analyzing social media trends to managing vast amounts of sensor data, big data applications are transforming how businesses operate and innovate. Here are some key examples that highlight the diverse uses of big data in different sectors:
Implementing best practices for big data is essential to effectively manage, analyze, and leverage large datasets. Adopting these practices ensures that data is accurate, secure, and utilized efficiently to drive meaningful insights and business outcomes. Best practices include establishing robust data governance, choosing the right technology stack, ensuring data quality, and maintaining privacy and security.
Additionally, organizations should focus on scalability, real-time processing, and ongoing monitoring to adapt to evolving data needs and technologies. By following these guidelines, businesses can maximize the value of their big data initiatives and enhance overall performance.
The concept of big data dates back to the early days of computing when data storage and processing capabilities were limited. Initially, data was stored on punch cards and magnetic tapes, and these technologies' limitations constrained analysis. In the 1960s and 1970s, the development of relational databases, like those from IBM, revolutionized data management by allowing for more efficient storage and querying.
The 1980s and 1990s saw significant advancements with the rise of data warehousing and online analytical processing (OLAP), which facilitated more complex data analysis and reporting. The term "big data" itself emerged in the early 2000s as digital technology began to increase, leading to an explosion in data volume and variety. The development of technologies like Hadoop and Apache Spark in the 2000s addressed the challenges of processing vast amounts of unstructured data.
With the advent of cloud computing and advancements in artificial intelligence (AI) and machine learning, big data analytics has become increasingly sophisticated, allowing organizations to derive actionable insights from massive datasets. Today, big data is integral to various industries, driving innovations in business intelligence, personalized experiences, and scientific research.
Big data has fundamentally transformed how organizations and industries operate by enabling the analysis of vast and complex datasets to uncover valuable insights. Its ability to process and analyze large volumes of structured and unstructured data has led to significant advancements in decision-making, operational efficiency, and innovation. From enhancing customer experiences and optimizing business processes to driving scientific discoveries and societal advancements, big data offers immense potential.
However, leveraging big data effectively requires addressing various challenges, such as ensuring data quality, maintaining security and privacy, and managing the scalability of data infrastructure. As technology continues to evolve, organizations must stay abreast of new tools and techniques while adhering to best practices for data governance and analytics. By overcoming these challenges and harnessing the full potential of big data, businesses and societies can achieve greater efficiencies, drive informed decisions, and foster innovation in an increasingly data-driven world.
Copy and paste below code to page Head section
Big Data refers to extremely large datasets that are complex and grow at an exponential rate. These datasets are so vast that traditional data processing tools cannot handle them effectively. Big Data involves collecting, storing, and analyzing large volumes of data from various sources to uncover patterns, trends, and insights that can drive decision-making and strategic planning.
Big Data provides organizations with valuable insights that enhance decision-making, improve operational efficiency, and drive innovation. By analyzing large datasets, businesses can identify trends, understand customer behavior, optimize processes, and gain a competitive edge. The insights gained from Big Data can lead to improved products, services, and customer experiences.
Managing Big Data comes with challenges such as high costs, data privacy and security concerns, data quality issues, and complexity in data management. Organizations may also face scalability issues and a shortage of skilled professionals. Addressing these challenges requires significant investment in technology and expertise to ensure effective data handling and analysis.
Big Data technologies include tools and platforms for data storage, processing, and analysis. Common technologies include Hadoop, Apache Spark, and NoSQL databases like MongoDB and Cassandra. These technologies help in managing large volumes of data and performing complex analyses to extract actionable insights.
Big Data differs from traditional data in terms of volume, velocity, and variety. While traditional data is usually structured and manageable with conventional tools, Big Data encompasses unstructured and semi-structured data that grows rapidly and comes from diverse sources. Big Data requires advanced technologies and methods to store, process, and analyze.
Organizations ensure data privacy by implementing robust security measures such as encryption, access controls, and regular audits. Compliance with data protection regulations like GDPR and CCPA is also crucial. It involves setting up secure data storage, managing user access, and ensuring that data handling practices meet legal and ethical standards.