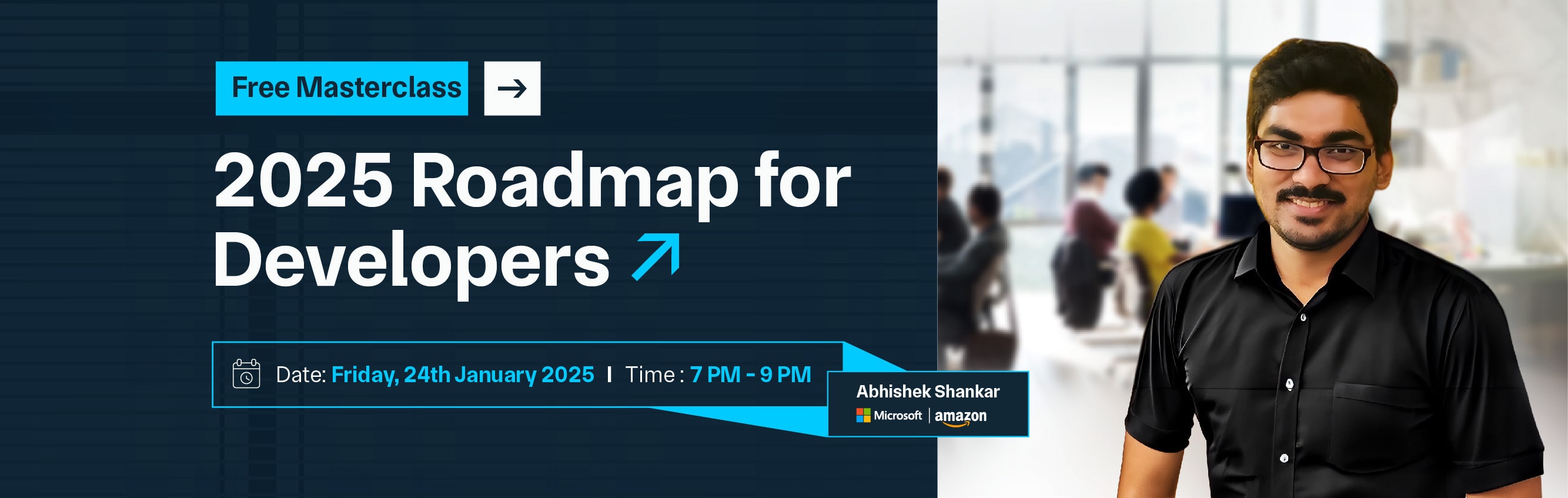

Data science is transforming healthcare by harnessing the power of data to improve patient outcomes and streamline medical processes. It involves analyzing large volumes of health data, such as electronic health records (EHRs), medical imaging, and genomic information, to uncover insights that drive better decision-making. Predictive analytics, a key component of data science, enables early disease detection and personalized treatment plans.
By examining patterns and trends in patient data, algorithms can forecast potential health issues before they become critical, allowing for proactive interventions. Machine learning models enhance diagnostic accuracy by identifying correlations in data that human clinicians might miss. Additionally, data science optimizes healthcare operations by improving resource allocation, reducing costs, and enhancing overall efficiency.
For example, predictive models can forecast patient admissions, helping hospitals manage staffing and equipment more effectively. Real-time data analysis also supports continuous patient monitoring and evidence-based decision-making, further advancing personalized care. As healthcare systems increasingly integrate data science, the potential to revolutionize patient care and operational efficiency continues to expand, marking a significant leap forward in the quest for better health outcomes.
Data science is transforming healthcare by analyzing vast amounts of data to improve patient care, enhance diagnostic accuracy, and streamline operations. It enables early disease detection, personalized treatments, and efficient resource management, leading to better health outcomes and more effective healthcare delivery. Data science is pivotal in healthcare for several compelling reasons:
Data science in healthcare drives innovation, improves patient care, and optimizes operational efficiency, making it an essential component of modern medical practice.
Data science is significantly impacting healthcare through various applications and use cases that enhance patient care, streamline operations, and drive medical advancements. Here are some key applications:
Predictive models analyze patient data to foresee potential health issues, such as predicting the onset of chronic diseases like diabetes or heart disease. For example, algorithms can identify patients at high risk for complications, enabling early intervention.
Data science tailors treatment plans based on individual patient data, including genetic information, lifestyle factors, and previous health records. This approach ensures treatments are more effective and aligned with each patient’s unique needs.
Machine learning algorithms process and interpret medical images, such as MRIs and X-rays, to assist in diagnosing conditions like tumors or fractures. These algorithms can detect abnormalities with high accuracy, often identifying issues that human radiologists may miss.
Data science accelerates drug discovery by analyzing biological data to identify potential drug candidates. Machine learning models can predict how different compounds will interact with biological targets, speeding up the development of new medications.
Real-time data analysis from wearable devices and remote monitoring tools helps track patient health metrics, such as heart rate and glucose levels. This enables continuous monitoring and timely interventions, improving the management of chronic conditions.
Data science improves hospital efficiency by optimizing scheduling, managing patient flow, and predicting resource needs. For example, predictive models can forecast patient admissions, helping hospitals allocate staff and resources more effectively.
Analyzing data across large populations helps identify health trends, manage outbreaks, and improve public health strategies. This application is crucial for understanding the spread of diseases and implementing preventive measures.
Data science provides clinicians with evidence-based recommendations and decision-support tools, enhancing diagnostic accuracy and treatment planning. Clinical decision support systems analyze patient data and medical literature to offer actionable insights.
Data science is revolutionizing healthcare by enabling more precise diagnostics, personalized treatments, and efficient operations, ultimately leading to improved patient outcomes and more effective healthcare delivery.
A data scientist in healthcare plays a crucial role in leveraging data to enhance patient care, optimize operations, and drive medical innovations. Here’s a detailed look at their key responsibilities:
Analyze large datasets, including electronic health records (EHRs), medical imaging, and genomic data, to extract actionable insights. This involves identifying patterns, trends, and anomalies that can inform clinical decisions and improve patient outcomes.
Develop and implement predictive models to forecast disease outbreaks, patient deterioration, or the likelihood of chronic conditions. These models help in early intervention and personalized treatment plans, improving patient management and preventive care.
Create visualizations and dashboards to present complex data in an understandable format. This helps clinicians, researchers, and decision-makers easily interpret data trends and make informed decisions based on the insights provided.
Design and deploy machine learning algorithms and artificial intelligence (AI) tools to enhance diagnostic accuracy and automate routine tasks. For example, AI can assist in interpreting medical images or predicting patient outcomes.
Work with medical researchers and practitioners to identify research questions and contribute to clinical studies. Data scientists help in designing experiments, analyzing results, and translating findings into actionable healthcare improvements.
Ensure the accuracy, completeness, and security of healthcare data. Implement data governance practices to maintain high standards of data quality and compliance with regulations like HIPAA (Health Insurance Portability and Accountability Act).
Analyze operational data to improve hospital workflows, resource allocation, and patient scheduling. Data scientists use predictive analytics to forecast patient admissions and optimize staffing levels, leading to more efficient hospital management.
Develop algorithms and models that support personalized treatment plans based on individual patient data, including genetic and lifestyle information. This ensures that treatments are tailored to each patient’s specific needs and conditions.
Analyze patient feedback and engagement data to identify areas for improvement in the patient experience. Data scientists work on strategies to enhance patient satisfaction and care quality.
Stay updated with the latest advancements in data science and healthcare technologies. Continuously adapt and refine methodologies to incorporate new tools and techniques that enhance data-driven healthcare solutions.
Data scientists in healthcare are essential for transforming data into actionable insights that improve patient care, enhance operational efficiency, and drive innovation. They work at the intersection of technology and medicine, using data to solve complex problems and advance the field of healthcare.
Data science offers numerous advantages in healthcare, enhancing both patient care and operational efficiency. Here’s a look at the key benefits:
Data science enables predictive analytics and personalized treatment plans. By analyzing patient data, healthcare providers can anticipate health issues, tailor treatments to individual needs, and implement preventative measures, leading to improved health outcomes and better management of chronic conditions.
Predictive models can identify patterns and risk factors from health data, allowing for early diagnosis of diseases like cancer, diabetes, and heart conditions. Early detection can significantly improve treatment success rates and patient survival.
Machine learning algorithms and data analysis tools assist in interpreting medical images and other diagnostic data with high precision. This reduces the likelihood of misdiagnoses and ensures more accurate and timely treatment.
Data science helps create personalized treatment plans based on individual patient data, including genetic information and lifestyle factors. This ensures that therapies are specifically tailored to each patient’s unique health profile, enhancing their effectiveness.
Analyzing operational data helps optimize hospital management, from patient scheduling to resource allocation. Data-driven insights improve workflow efficiency, reduce wait times, and enhance overall hospital operations.
By identifying inefficiencies and predicting patient needs, data science helps reduce unnecessary tests and hospital readmissions. This leads to significant cost savings for both healthcare providers and patients.
Real-time data from wearable devices and remote monitoring tools allows continuous tracking of patient health metrics. This enables timely interventions and adjustments to treatment plans based on real-time data.
Data science provides healthcare professionals with actionable insights and evidence-based recommendations. This supports more informed and effective decision-making, improving overall care quality.
Data science accelerates medical research by analyzing large datasets to uncover new insights and trends. This drives innovation in drug development, treatment methods, and understanding of diseases.
By analyzing patient feedback and engagement data, healthcare providers can identify areas for improvement in patient care and service delivery, leading to a better overall patient experience and increased satisfaction.
Data science is revolutionizing healthcare by transforming how data is used to improve patient care, streamline operations, and drive medical research. By leveraging advanced algorithms and analytics, healthcare organizations can enhance diagnostics, personalize treatments, and optimize resource management for better health outcomes.
Data science tools are crucial in transforming healthcare through advanced analytics, improving patient care, and optimizing operations. Here are some key tools used in the healthcare industry:
A versatile programming language widely used for data analysis, machine learning, and statistical modeling. Python libraries such as Pandas, NumPy, and Scikit-Learn are particularly popular for handling and analyzing healthcare data.
A statistical programming language is known for its powerful data visualization and statistical analysis capabilities. R is commonly used for bioinformatics, epidemiological studies, and clinical trial analysis.
A language used for managing and querying relational databases. SQL is essential for extracting and manipulating healthcare data stored in databases like electronic health records (EHRs).
A data visualization tool that helps in creating interactive and shareable dashboards. Tableau is used in healthcare for visualizing patient data, tracking performance metrics, and enhancing decision-making.
An open-source framework for processing and storing large datasets across distributed computing environments. Hadoop is used in healthcare to handle big data, such as genomics and large-scale patient records.
A software suite used for advanced analytics, business intelligence, and data management. SAS provides robust tools for statistical analysis and predictive modeling in healthcare research and clinical trials.
An open-source machine learning framework developed by Google. TensorFlow is used for building and training deep learning models, such as those used for medical image analysis and predictive analytics.
A unified analytics engine for big data processing with built-in modules for SQL, streaming, and machine learning. Spark is utilized in healthcare for real-time data analysis and large-scale data processing.
An open-source data analytics platform with a graphical interface. KNIME is used in healthcare for building data pipelines, performing statistical analysis, and developing machine learning models.
A cloud computing platform offering a range of services, including machine learning, data storage, and analytics. Azure provides scalable solutions for managing and analyzing healthcare data in the cloud.
A suite of AI and data analytics tools designed to improve healthcare delivery. IBM Watson Health offers solutions for data integration, clinical decision support, and personalized medicine.
These tools enable healthcare organizations to harness the power of data, leading to improved diagnostics, personalized treatments, and efficient healthcare delivery.
Becoming a healthcare data scientist in 2025 involves a combination of specialized education, technical skills, and domain knowledge. Start by obtaining a relevant degree, such as in data science, statistics, computer science, or a related field. Advanced degrees like a master’s or PhD can be advantageous. Develop expertise in programming languages like Python and R and become proficient in data manipulation tools and techniques.
Understanding healthcare data specifically requires knowledge of medical terminology, health informatics, and regulatory issues such as HIPAA. Practical experience is crucial, so seek internships or work opportunities in healthcare settings where you can apply data science principles to real-world problems.
Additionally, staying current with emerging technologies and trends in both data science and healthcare will help you remain competitive in this evolving field. Networking with professionals and joining relevant organizations can also provide valuable insights and opportunities.
The future of data science in healthcare is poised for transformative advancements, driven by continuous technological innovations and the increasing complexity of healthcare data. Here’s a glimpse into what lies ahead:
The future of data science in healthcare holds remarkable potential for transforming patient care, operational efficiency, and medical research. As we advance, the integration of sophisticated analytics, machine learning, and real-time data will drive more personalized and proactive healthcare solutions. Embracing these innovations will enable healthcare professionals to make better-informed decisions, optimize treatment plans, and improve patient outcomes.
Continued advancements in data science promise to address current challenges and unlock new opportunities for enhancing the overall quality and accessibility of healthcare. As we move forward, the collaboration between technology and healthcare will be crucial in shaping a healthier future for all.
1. Invest in Infrastructure and Technology
2. Foster Data Integration and Interoperability
3. Enhance Data Quality and Governance
4. Promote Collaboration and Knowledge Sharing
5. Focus on Talent Development and Training
By implementing these strategies, the healthcare sector can effectively advance its use of big data and data science, leading to more personalized care, efficient operations, and better health outcomes.
Advancing big data and data science in healthcare offers transformative benefits. By enhancing data processing capabilities, integrating diverse data sources, ensuring high data quality, fostering collaboration, and developing skilled professionals, the healthcare sector can achieve more accurate insights, improve patient care, and drive innovation.
Advancing big data and data science in healthcare is crucial for transforming patient care and operational efficiency. By investing in technology, integrating data sources, ensuring data quality, promoting collaboration, and developing talent, the healthcare industry can leverage comprehensive insights and innovative solutions.
These advancements will lead to more personalized care, better decision-making, and improved health outcomes, ultimately shaping a more effective and responsive healthcare system for the future.
Copy and paste below code to page Head section
Big data in healthcare involves analyzing large and complex datasets from various sources, such as electronic health records, medical imaging, and wearable devices. This analysis provides insights that can improve patient care, optimize operations, and drive research and innovation.
Data integration consolidates information from different sources, offering a comprehensive view of patient health and operational metrics. This integration improves care coordination, enables more accurate insights, and enhances decision-making by providing a holistic perspective.
High data quality ensures that the analyses and insights derived from data are accurate and reliable. This leads to more effective interventions and strategies while protecting patient privacy and ensuring compliance with regulations, which builds trust in the data-driven decision-making process.
Collaboration between healthcare providers, researchers, and technology companies fosters the exchange of knowledge and best practices. This collaborative environment accelerates innovation, leads to the development of new solutions, and improves the overall effectiveness of data science applications in healthcare.
A healthcare data scientist should possess skills in programming (e.g., Python, R), statistical analysis, data visualization, and machine learning. Additionally, understanding healthcare systems and regulations, as well as having strong problem-solving abilities, is crucial for applying data science effectively in the healthcare sector.
Investing in advanced data infrastructure, such as cloud storage and high-performance computing, enhances the ability to handle large volumes of data efficiently. This investment supports faster data processing, real-time analysis, and scalability to accommodate growing data needs and evolving technologies.